Best Practices and Ethical Considerations in AI-Powered Medical Imaging with ALAFIA’s Camilo Buscaron
Episode Overview
Episode Topic: In this episode of Skeleton Crew – The Rad Tech Show, we sit down with Camilo Buscaron, founder of ALAFIA, to discuss the innovative field of omics and its integration with advanced computing technologies. Camilo introduces the concept of Omics Computational Technology Solutions, highlighting how these solutions are transforming the way biological data is processed and analyzed. He explains the various types of omics, such as proteomics and glycomics, and their significance in precision medicine. The conversation provides a comprehensive overview of how high-performance computing is being leveraged to enhance medical research and healthcare outcomes.
Lessons You’ll Learn: Listeners will gain valuable insights into the cutting-edge field of omics and its impact on healthcare and research. Camilo Buscaron shares his expertise on omics computing solutions, illustrating how these solutions are crucial for handling the vast amounts of data generated in biological studies. Listeners will learn about the importance of optimizing software and hardware to achieve higher performance in medical applications. The episode also covers the practical applications of these technologies in radiology and pathology, demonstrating how they can significantly speed up data processing and improve diagnostic accuracy.
About Our Guests: Camilo Buscaron is a seasoned expert in high-performance computing and the founder of ALAFIA, a company at the forefront of Omics Computational Technology Solutions. With a career spanning over 15 years, Camilo has been instrumental in developing advanced computational technologies for robotics and autonomous vehicles in Silicon Valley. His work has now pivoted towards the medical field, where he is pioneering the use of high-performance computing to enhance precision medicine and biological research. Camilo’s experience and innovative approach make him a leading figure in integrating omics with computational technology.
Topics Covered: Throughout the episode, we cover a range of topics related to omics and computational technology. Key discussions include the definition and importance of omics in biological research, the development of omics computing solutions, and their application in precision medicine. The conversation also elaborates on the challenges and solutions in optimizing software for high-performance computing, particularly in medical imaging and digital pathology. Listeners will also hear about the collaborative efforts to standardize data formats in tomography and the future potential of these technologies in revolutionizing healthcare and medical research.
Our Guest: Camilo Buscaron- Leading Omics Computational Technology Solutions for Precision Medicine
Camilo Buscaron is the founder of ALAFIA, a company at the forefront of developing Omics Computational Technology Solutions for precision medicine and biological research. He is a pioneering figure in the field of high-performance computing and artificial intelligence, with a career that spans over 15 years. Before founding ALAFIA, he led significant technological advancements at major corporations such as Amazon Web Services (AWS) and NVIDIA. At AWS, he served as the Chief Technologist for Robotics and Autonomous Systems, while at NVIDIA, he was the Lead System Engineer, focusing on computational technologies for autonomous vehicles and AI-driven applications
Camilo’s expertise is rooted in a deep understanding of various advanced technologies. He has been instrumental in developing software engineering teams, leading product design, and managing global engineering teams across multiple disciplines. His technical proficiency includes areas such as artificial intelligence, deep learning algorithms, robotics, and image processing. These skills have been critical in his work at ALAFIA, where he integrates high-performance computing with biological data to create solutions that accelerate medical research and improve healthcare outcomes.
Beyond his professional achievements, Camilo is committed to educating the next generation of biologists and technologists. He collaborates with academic institutions and research organizations, aiming to advance the field of computational biology. His efforts include working with the International Society for Computational Biology to provide tools and resources that enhance the capabilities of bioinformaticians and medical researchers. Camilo’s vision is to enable significant breakthroughs in precision medicine by leveraging Omics Computational Technology Solutions to analyze and interpret complex biological data more effectively.
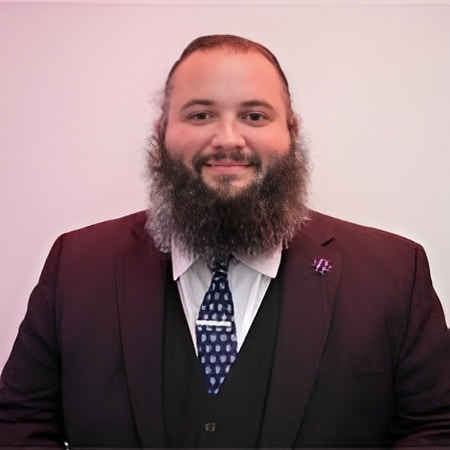
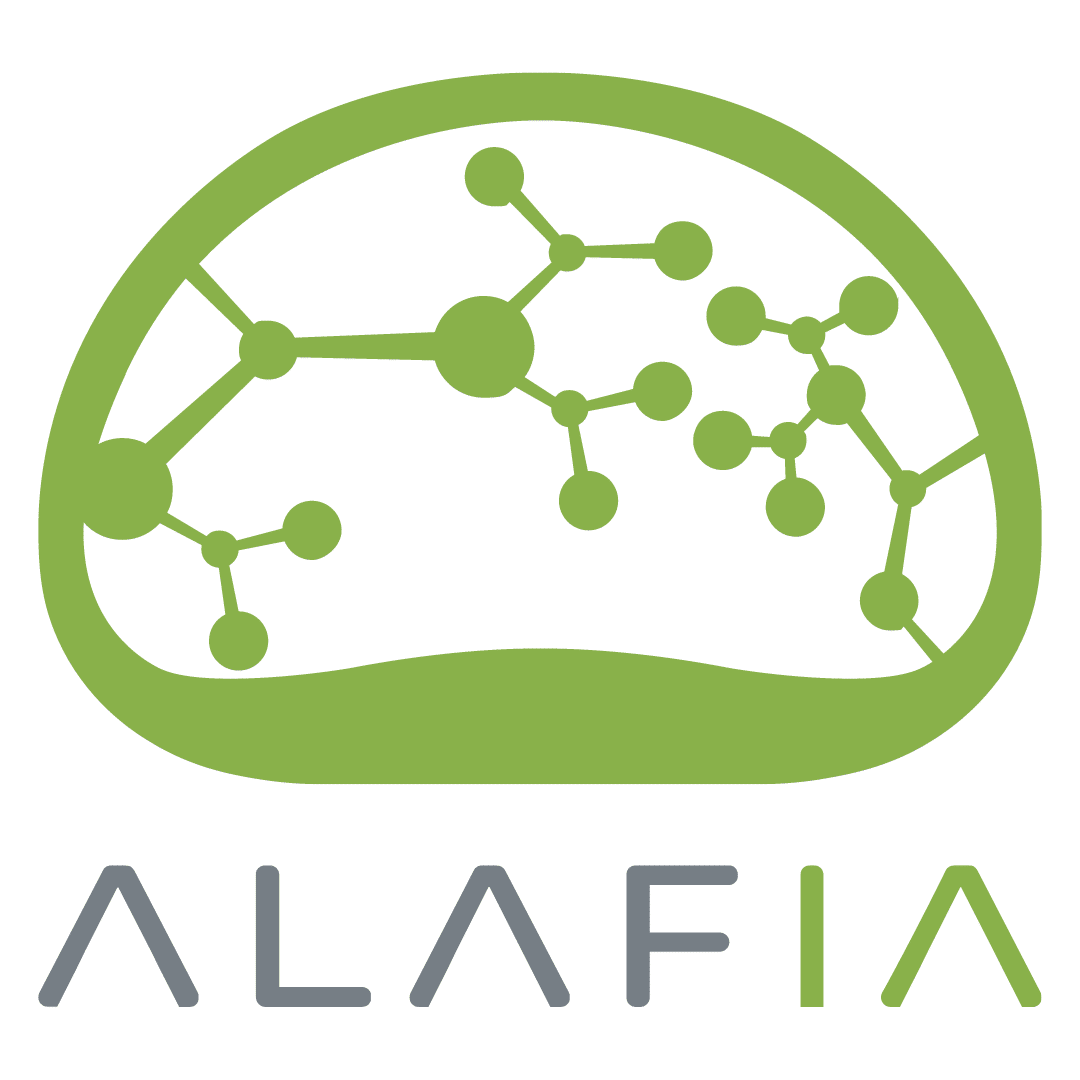
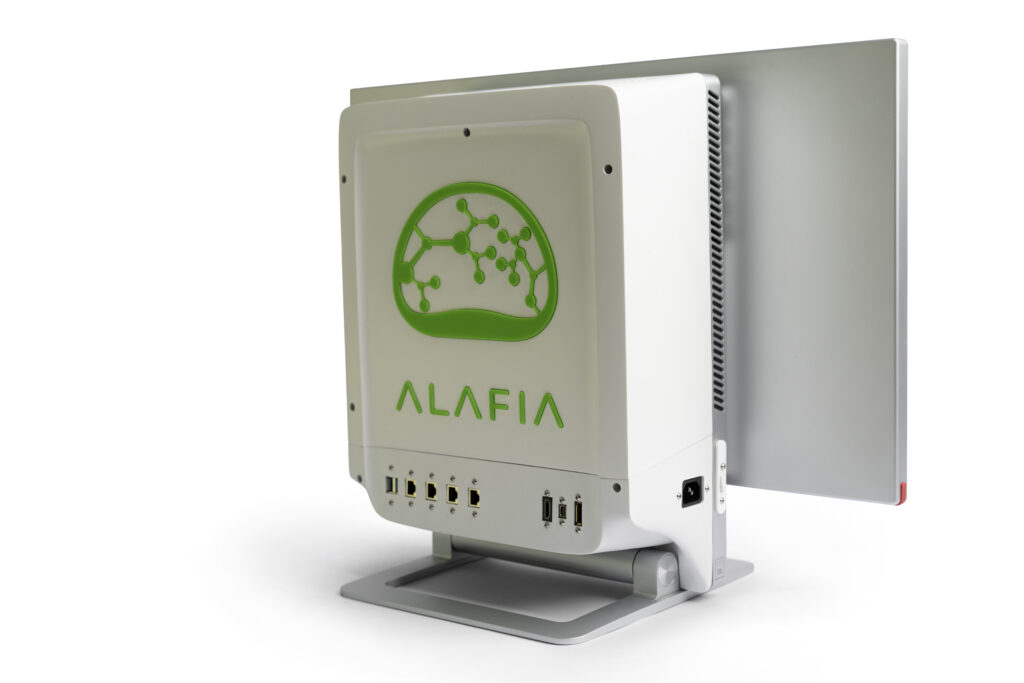
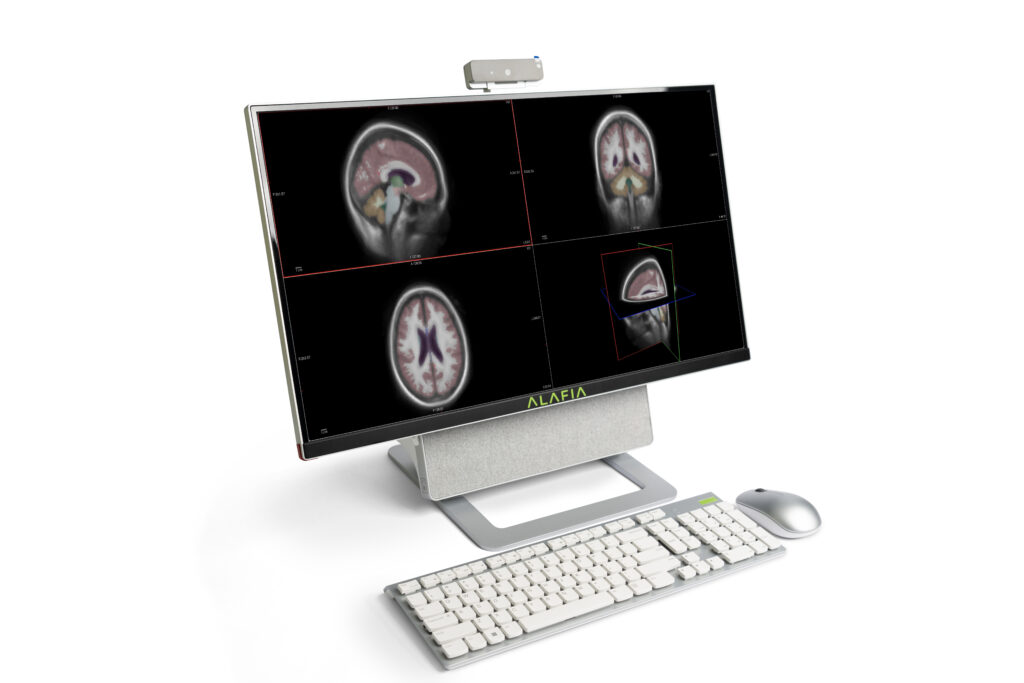
Episode Transcript
Jennifer Callahan: Hey, everybody, welcome back to another episode of The Skeleton-Crew. I’m your host, Jen Callahan, and today I have a guest with me. His name is Camilo Buscaron, and he is the founder of a company out there called ALAFIA. They’re doing some great stuff out there, I’ve never heard of this, but it’s called omics computing solution. So this is going to be news for you and news for me, myself, and learning about something new as I just said. So I’m going to let Cam go ahead and take the ball. Then Cam, tell us about ALAFIA and then maybe give us a little background of yourself and how you have ventured into your new company.
Camilo Buscaron: Thank you, Jen. A pleasure to be here with you today. I think it’s great that you mentioned the omics computing part because that’s something that I think biologists, for the most part, understand what omics is which is the essential life data that comes out of all the types of biological samples that you can get. Sort of the broad term, but then it can be proteomics glycomics. There’s a whole field of omics computational or biological analysis. When we introduce the term computing, what we mean by that is we believe that we can adapt the computational data structures and the computational technology that we use in a regular computer to be better suited for the biological processes and the biological ultimately the data structures, how we would encode that in a computer that comes from that technology. So, thank you for bringing that part up. Then a bit about me I spent about 15 years building robotics technology.
Camilo Buscaron: Most of my career has been building robotics technology. I got to work in some great startups and major corporations in Silicon Valley that were developing mostly computational technology for autonomous vehicles. So we’re building computers, high-performance computers for autonomous vehicles. We’re building APIs for robotics simulations, and we’re building robots themselves. We ultimately ended up building a lab because we had developed some computer vision technology that was becoming very popular among medical imaging researchers. We had this technology that we developed for general purpose, and there were quite a bit of a number of medical imaging researchers using our technology, publishing papers on how they were using it. One thing led to the next. We ended up at a hospital network, and they wanted to build an application using the library we had created. We walked in there, we realized their problem wasn’t that they needed AI. They had just underinvested in their infrastructure. So that led us to build the products that were launching this summer.
Jennifer Callahan: Okay, so let’s talk about a little bit of the different products. I’ll let you go through them and name them because some of them have a bunch of different numbers and some letters attached to them. Maybe you could give more insight as to why they’re named that way, and then maybe if it correlates to what it possibly does. But I know that I saw at least two, possibly three of the different products that you have coming out.
Camilo Buscaron: Yes. So the main product that we’re building is a platform for precision medicine. It’s ultimately an all-in-one high-performance computer. But we have optimized every layer of the software stack to perform at a significantly higher level bar than you would would with a regular computer. So we’ve taken sort of the most powerful computer you can find in the world today, sort of off the shelf. We have integrated it into our system. And then we optimized every layer of the software stack from the kernel to the operating system to the device drivers to the middle, middle to the middleware. Normally our customers typically have a scientific pipeline. it could be a neurology research pipeline. It could be a computational biology pipeline, and somehow it doesn’t perform great in the existing systems that they could buy. So what we do is, we help them optimize our software to leverage the hardware architecture and perform the best that it can perform in any environment. So a lot of our, a lot of our customer engagements today involve very hands-on developing with our customers to make their software. highly more performant, leveraging our architecture.
Jennifer Callahan: Okay. Now, is it possibly because the software that they have is older and they just like this is kind of just giving a little bump, or is it because it’s just completely lacking something?
Camilo Buscaron: It’s usually a mix of things. So researchers typically are not software engineers. So they don’t develop optimized software from the beginning. So that’s usually one setback. Other areas could be that they’re not as familiar with high-performance computing and how you can integrate, how you can leverage computational elements sort of matrix, let’s say, matrix multiplication intrinsics or something or an instruction called SIMD, single instruction multiple data. This is a well-known computational element for supercomputing, but medical researchers may not be familiar with sort of the computing the edge computing capabilities that you could leverage in a high-performance system. So helping them become aware of that and making their data structures more seemly friendly than being able to vectorize those computations adds a significant speed up. We also have to scale out computational capability. So if they if they have a piece of software that can be multi-threaded we have our systems go from 128 cores to 256 cores. So they can leverage that compute in sort of an optimized software. Software stack. They’re able to gain a significant advantage that they wouldn’t from a normal system.
Jennifer Callahan: All right. Are you hooking this up, directly to say, a CAT scan machine? They’re like a processing station or control station, I guess I should say, or like the x-ray control station or is it something that’s more on the back end of where the radiologists are reading?
Camilo Buscaron: Yes. So this is more on the radiology reading side or on the pathologist side. So, getting data from a CAT scan usually would happen through a PAC system. So the data goes from the scanning systems through a pack. Then we receive that data and then we can process that data. So if if there are applications that are cutting edge and need to run AI algorithms we’re very good at that. We can we can run that very well. Another perfect example that it’s readily available that we’re working with some customers on is digital pathology. So digital pathology traditionally, when a pathologist looks at a tissue sample or a blood sample, they put it on their microscope and analyze it. Well, that doesn’t happen anymore. Today there are these big scanner machines multi-million dollar scanner machines, similar to a CTA or a PET scan. The pathologist would put the the tissue or the blood sample which would get scanned by the machine. The output of that machine is a very large image file.
Jennifer Callahan: This episode is brought to you by xraytech.org, the Rad Tech Career Resource. If you’re considering a career in radiology, check out xraytech.org to get honest information on schools, degree options, career paths, and salaries.
Camilo Buscaron: One of the differences between pathology and radiology is that a pathologist can read the way, uh a radiologist would a scan you need to be able to run more tools to, you know classify cells, count the number of cells to see if there are any elements of cancer or being able to quantify how spread out a cancer cell may be. So they use a piece of software that does a lot of pre-processing. Some of these workloads are things that run overnight and take multiple hours. So where we add value is accelerating these workloads and typical workloads that would take maybe 30 minutes or 40 minutes in a regular computer today. We can do them under 30s. So it’s different. It’s different than a radiology looking at a lesion in a scan. But I think with the advent of AI you have more tools. in radiology to like long nodules and long nodules detection. Those are very hard things for a radiologist to do. That could be easily missed. Because in 400 frames, you may have 3 or 4 frames that show you a long nodule. That’s where somewhere where an AI artificial intelligence application could help in our system would relatively support that type of application.
Jennifer Callahan: Sure, because it can sort through it easier than radiologists right now. Is this best utilized for a certain type of scan? More so than others?
Camilo Buscaron: It all depends. The applications that our customers are looking to process like I said earlier, is just a very powerful computer, probably the most powerful computer you could buy in the world. Then it’s a matter of what software application they use today. And if there are some limitations, typically the customers that we engage on, they’re not very happy with the existing systems that they have available to run some of these workloads.
Jennifer Callahan: Now is this something that the scans are automatically run through, or is it something that the radiologist has to choose for it to go through?
Camilo Buscaron: Right. So typically with a PAC system, we don’t dictate which PAC or customers should use. However, PAC systems have different features in how they prioritize readings. What work in what case goes to a radiologist? That all depends on sort of the PACs that our customers will be using. But in cardiology use cases there are great tools for that. Those tools in sort for cardiology use cases do run a lot of computations to measure the flow of blood, not just imaging, but running computations to calculate flow and other elements of cardiological analysis.
Jennifer Callahan: Now I was looking on your website and I saw there were many different images of body parts from scans that look very different than, say, images that I’ve seen from scans I don’t know if you have anything you might possibly be able to share for those who are watching us on YouTube. But there was one of a cross-section of the heart. But the the image of the heart itself was very different than probably it would be seen on a CAT scan. Is that something that I’m assuming that this technology is doing, that transforming the way that it’s viewed?
Camilo Buscaron: Yes. So there is one major use case that we support with a number of customers, which is reconstruction. The way reconstruction happens and this could be, let’s say, there’s cortical reconstruction. So let’s say reconstruction from MRI images of the brain or fMRI images where you have 2D images, you have maybe 400 or 500 2D image slices. Then there’s a piece of software that is used to reconstruct those 2D images and being able to create a 3D mesh of the organ. So this is very common in neurology research for cortical reconstruction. Tomography is another area where you also have to be able to reconstruct the images. Then there are a number of software tools. Those types of workloads are heavily computationally intensive. They take a significant number of algorithms to preprocess and compute that reconstruction as accurately as possible from the 2D images that are the elements that you see on the website there. 3D images generated from 2D scans.
Jennifer Callahan: Okay, cool.
Camilo Buscaron: And that is somewhere where the field is moving towards even, even standardizing how this data comes off the machines. We’ve supported a number of researchers sort of pioneering sort of this collaborative effort to standardize how the data formats and the images come out of tomography systems to be able to reconstruct them. Sort of what DICOM did for radiology as a standard format for viewing. There are now efforts to standardize tomography data to be able to reconstruct that data and look at it as 3D meshes of an organ or a scan.
Jennifer Callahan: Okay. So obviously this is very intricate stuff that you’re doing. Have you met challenges along the way while developing your different software?
Camilo Buscaron: Yes. A significant number of efforts to be able to get every aspect of the pipeline working. One of them has been developing the hardware technology that can perform these workloads. That can run these workloads. So that has been an effort we have been pursuing for about a year. We’re starting to ship our hardware technology in our product. And then there’s significant software migration for existing applications because we sort of have a new microarchitecture that’s part of our strategy into being able to create a powerful computer, the form factor and power envelope that we have a microarchitecture that traditional computers haven’t used. So there’s a significant effort. There’s depending on what the application is, there’s an effort to migrate that software to fit our microarchitecture. But by doing so, then we leverage the additional compute, and we’re able to increase the performance of the application. So, so that is the benefit. There’s some level of work involved. But once we do that, then we’re able to gain significant advantage on behalf of our customers ultimately. Most of the applications that we migrate for our customers depend on the use cases that they need.
Jennifer Callahan: Do you have this currently integrated into a fair amount of health systems?
Camilo Buscaron: Not quite health systems for say. Most of our customers today today are on the research side. We have a number. We do have clinics. We have a number of clinics performing neurology research. We have a research center associated with Massachusetts, with Mass General. So that per se, but it’s mostly on the research side for different use cases that involve neurology research, like transcranial magnetic stimulation where they use a lot of computation to figure out how to place the coils to stimulate the brain. For cases like depression and obsessive-compulsive disorders, there have been found significant improvements there because there’s supposed to be a pharma pharmacological approach, but there are limited side effects. So a number of more research are our most of our customers today are bioinformaticians. They’re sort of the healthcare application developers. They’re figuring out what solutions to provide to physicians and what they’re learning. What they like about our system is that they develop their solution or our system, then our system is also something that can be on the desk side of a doctor as opposed to being able to create something that would run on the cloud over, and over a long period of time. Our system can be a distribution method for them to deploy their applications. So mostly bioinformaticians, and that sort of use case.
Jennifer Callahan: Yes. Well, you mentioned Mass General. Massachusetts General for those that are listening. Then I also saw that you are partnering with Shriners, I guess, again, for the research aspect of it.
Camilo Buscaron: Correct. So Shriners is completely focused on orthopedics. That’s an element where 3D reconstruction and 3D applications need to manipulate 3D orthopedic solutions like a knee joint or you want to simulate how a knee would behave under different materials if you use different support materials, those are very three heavy graphical have significant graphical elements that are used to our system for visualization because we sort of have a great sort of interactive 4K screen. All those graphical elements that are 3D structures that need to be visualized and simulated are use cases that we fit a solution very well.
Jennifer Callahan: All right. So obviously you have a lot going on here in terms of the research world and you’ve developed some great products, where do you see yourself heading in the future with the company? Do you guys have something on the like in the works that you’re hoping to launch along with the other products?
Camilo Buscaron: Yes, we’re very excited. To continue to develop the solutions for Bioinformaticians. We’re partnering with the International Society for Computational Biology. Okay. This is mostly around intelligent systems for computational biology. Mostly to educate the next generation of biologists. Now biology has become a field that is not just a science that you study, it’s an engineering field where you can create and create tools and experiments that are very valuable. So ultimately they they are the developers that are going to make the application for the doctor. So we hope to provide them with the tools that help accelerate their discoveries and research. So we’ll be very happy that some major breakthroughs just happen to have happened in our system. That’s sort of our goal and our vision.
Jennifer Callahan: Yes. Doing great things. Everybody today, this is Camilo Buscaron with me talking about the omics computer solutions that they’re offering out there in the medical world. So, Cam, thanks for taking the time to be with me today and sharing everything that your company is doing, looking forward to hearing about the new developments that you’ll have coming out in the future. So everybody, Jen Callahan and Cam this week with the Skeleton-Crew, you can check us out on YouTube, Apple Podcasts, and Spotify. So thanks for being with us today, Cam. Thanks again for your time, I appreciate it.
Camilo Buscaron: Thank you, Jen. Thank you folks. Have a great day.
Jennifer Callahan: All right. See you, everybody. You’ve been watching the Skeleton-Crew brought to you by xraytech.org on the next episode, join us to explore the present and the future of the Rad Tech career in the field of radiology.