Tech Ventures to Medical Breakthroughs with Joshua Miller of Gradient Health
Episode Overview
Episode Topic: In this episode of Skeleton Crew – The Rad Tech Show, we delve into the intersection of AI and healthcare with Joshua Miller, the CEO of Gradient Health. The conversation unfolds to explore the pivotal role Gradient Health plays in shaping AI development by providing labeled datasets for disease detection. Miller’s expertise in electrical and computer engineering adds a unique perspective to the discussion, shedding light on how technology is transforming medical diagnostics and treatment through innovative data analysis.
Lessons You’ll Learn: Listeners gain valuable insights into Miller’s leadership journey, drawing parallels between his experiences at FarmShots and Gradient Health. The episode unfolds lessons learned from promoting AI in healthcare, showcasing the challenges and triumphs encountered along the way. Listeners can expect to glean practical advice for aspiring entrepreneurs in the medical technology field, grounded in Miller’s extensive background and success in the tech industry.
About Our Guests: Joshua Miller, the featured guest in this episode, is the CEO of Gradient Health, a company pioneering advancements in AI-driven healthcare. With a background in electrical and computer engineering, Miller brings a wealth of experience to the podcast. Having led successful ventures like FarmShots, his journey reflects a commitment to pushing the boundaries of technology to enhance medical diagnostics and treatment.
Topics Covered: Dive into the rich tapestry of topics covered in this episode, starting with Gradient Health’s crucial role in providing labeled datasets for AI development in healthcare. Miller shares insights into the challenges and triumphs of promoting AI in the medical field, drawing on his leadership experiences. The conversation delves into the future outlook of AI in healthcare, providing listeners with a glimpse into the transformative potential of technology in the medical landscape. Aspiring entrepreneurs in the medical technology field can expect practical advice and encouragement from Miller’s experiences.
Our Guest: Joshua Miller – Transforming Healthcare Through AI Innovation
Joshua Miller, the CEO of Gradient Health, is a visionary entrepreneur with a background in electrical and computer engineering. A graduate of Duke University, Miller’s journey into entrepreneurship began when he crossed paths with Ouwen Huang, his co-founder for their first successful venture, FarmShots. Unlike his peers who were pursuing internships at major tech companies, Miller’s curiosity led him to a NASA research paper from the ’60s that explored the use of satellite images in analyzing crop performance.
The realization that advancements in satellite technology had occurred since the paper’s publication sparked the idea for FarmShots. Miller and Huang seized the opportunity to leverage satellite imagery to provide valuable insights into crop health for farmers and agronomists. Their initiative gained traction as they reached out to potential customers, discovering a strong demand for the services they could offer. FarmShots quickly became a success story, expanding its reach globally, with customers spanning as far as New Zealand.
Building on the success of FarmShots, Miller’s entrepreneurial spirit persists in his role as the CEO of Gradient Health. With a passion for technology and a commitment to making impactful contributions to the field of healthcare, Miller continues to lead innovative ventures that push the boundaries of what’s possible at the intersection of technology and medicine.
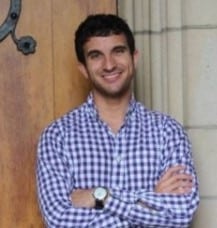
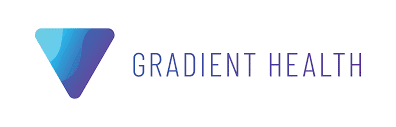
Episode Transcript
Joshua Miller: So what we’re seeing specifically in that space is not saying, hey, there’s a stroke in this case, it’s saying, okay, radiologist, you’ve got a thousand studies you might be looking at in that day. This looks like it might have a stroke. I’m going to move that to the top of the stack, because that’s the most important thing for you to look at. Whereas the guy with a broken toe can maybe afford to wait.
Jennifer Callahan: Welcome to the Skeleton Crew. I’m your host, Jen Callahan, a technologist with 10+ years of experience. In each episode, we will explore the fast-paced, ever-changing stuff that’s the completely crazy field of radiology. We will speak to technologists from all different modalities about their careers and education, the educators and leaders who are shaping the field today, and the business executives whose innovations are paving the future of radiology. This episode is brought to you by xraytechnicianschools.com. If you’re considering a career in X-ray, visit xraytechnicianschools.com to explore schools and to get honest information on career paths, salaries, and degree options. Hey, everybody, welcome back to another episode of The Skeleton Crew. My name is Jen Callahan here again, and tonight I have a great guest with me. His name is Josh Miller. He is the CEO of an awesome company called Gradient Health. And I’m going to leave all the important information for him to share. Um, instead of me trying to jumble through it because it’s really important, groundbreaking work that he’s doing. But I’m looking at all the words I have in front of me. And I said to him, I said, I think I’m just going to leave it up to you to share what your awesome company does.
Joshua Miller: Thanks for having me, Jen. Yeah. Quick background on Gradient Health is, look, we’ve all kind of seen the buzz around AI and radiology. If you want to build an AI in radiology, let’s say something like a mammography AI that can detect breast cancer. To build that AI, you need tens of thousands of examples of what breast cancer looks like to teach that AI. So we’re a company that what we do is we deliver those kinds of data sets for folks that are building AI in this space. We’re one of a few places in the world you can go to and say, look, I need 100,000 de-identified mammograms to train an AI, right? I’m just using mammography as an example, but we have all kinds of radiology data anonymized that we make available for this kind of research.
Jennifer Callahan: All right. So my first question when I was looking through your website was, how do you actually come to have these images to be able to put into these data sets?
Joshua Miller: Yeah. So we mainly work with hospital systems around the world, right? What we do is we de-identify the information, so we remove all of the doctor’s notes, and any kind of patient identifying information, and then we de-identify the imaging itself. So sometimes you’ll see like annotations, you know, where the Rad tech wrote something in. We’ll remove all of those. So that way the data can no longer be connected to the patient. We then pull that data into our system and make it searchable. That means our customers are the AI companies, also the radiology equipment manufacturers, and even some pharmaceutical companies that are all in this race to detect disease early.
Jennifer Callahan: Okay, so I’m going to go even further back to that. Do you go to health care systems? You know, do you contact them and say, “I’m in this business looking for images to be able to help AI developers, would you be willing to allow me to access your images? we’ll make them anonymous.” Is that kind of how it goes?
Joshua Miller: Yeah. And the majority of the systems we work with are either community hospitals, right, or private radiology clinics. And kind of our philosophy on that is we want to make sure those hospitals are using that money for innovation and for patient care. So that way, you know, any kind of data usage, the value that’s created from that goes back to the patient population themselves.
Jennifer Callahan: Okay. So you’re paying them to be able to access the images.
Joshua Miller: We do either a grant back to the hospital or a revenue share if that data gets used in research, etc.
Jennifer Callahan: So, another thing while I was on the website looking, is that talking about clients being able to have access to diverse images. So obviously certain diseases and medical conditions are more prevalent in certain ethnicities. When you’re looking at these different images to set up these data sets, are you looking for a specific ethnicity or an age bracket, or even a gender?
Joshua Miller: All of the above and more. Right? Diversity when it comes to radiology, data has like ten different dimensions. It’s not just ethnicity. It’s things like BMI, things like the manufacturer, your eye to only work on Siemens machines, then you can’t really expect it to work on a GE machine. Really. We think about diversity. Yes, in terms of age, in terms of sex, in terms of ethnicity, but also in terms of BMI, slice thickness, age of the machine, the manufacturer, the model, even regional variation, like a Caucasian patient in a rural town is very different from a Caucasian patient in a metropolitan area. And so you need to have that level of diversity captured as well. That’s kind of the challenge in this space is if you build an AI and you train it only on. You know, white guys from New York, you can’t expect it to work for every other patient. And so one of the fundamental problems we solve is making sure that all that representative data is put into training that model. So that way the AI can answer questions about everyone equally. And we’re not exacerbating unequal health care. We’re making it more equal.
Jennifer Callahan: What different companies do you have contacting you in terms of being able to access your data set? Obviously, they’re developing AI, but I guess really more like pharmaceutical companies, or maybe they’re private companies or are looking to branch into treatment for certain diseases?
Joshua Miller: I would say the majority of the folks we work with are AI companies that are making radiology. I need a bunch of data to train it, and you’ve got the like equipment manufacturers, the GE, Siemens, Philips, those guys, they’re all trying to build AI as well. And they need data. You’ve even got the med-device companies, right? The folks who make hardware trying to build radiology AI. You’ve got the pharmaceutical companies trying to identify, you know, disease early, either to get people on certain treatments and bring some value to the patient or to even recruit for clinical trials. And then, interestingly enough, universities themselves are a good part of our customer base, right? So if you’re a researcher at XYZ University and you’re trying to build something as part of a grant, you typically need access to this kind of data. There are really five categories of folks that we work with, but we’ve seen some really, really cool use cases that when I started this company, I would have never imagined.
Jennifer Callahan: Do you find that? Is it solely like more like cancer-driven in terms of treatment, and pre-treatment, or are there other medical conditions that are kind of as prevalent as cancer?
Joshua Miller: That’s a great question. Like maybe, maybe a way to put that is, you know, what are people building these algorithms for? It’s almost 40% oncology. So a large portion of it is oncology. 40% of it is neurology. So, finding stroke hemorrhage kind of things that are very time sensitive where you want to find them quickly. And then the kind of tail end 20% is everything from MSK to, you know, um like longitudinal analysis, population health, things like that. But really oncology and neurology are kind of the two, let’s say, the largest fields people are doing this research in.
Jennifer Callahan: I’ve heard that sometimes a stroke doesn’t show up on a CAT scan immediately. Like, it’s almost like it’s like a day later. And really, what they’re relying on to diagnose a stroke at that point is really like symptoms. Maybe if there is something minor on the image that is seen on ones that have been taken later, that can be used at the time the patient’s coming in to be scanned immediately.
Joshua Miller: Yeah. And there are certain patterns that an eye can see if you’re talking about like small values of pixels, like the window leveling being a little different on a CAT scan, the eye can pick up on that right in the way a human I can’t. And so what we’re seeing specifically in that space is not saying, hey, there’s a stroke in this case, it’s saying, okay, radiologist, you’ve got a thousand studies you might be looking at in that day. This looks like it might have a stroke. I’m going to move that to the top of the stack. Right. Because that’s the most important thing for you to look at. Whereas the guy with a broken toe can maybe afford to wait.
Jennifer Callahan: You’re doing this as a startup company, but this isn’t really your first startup. It seems like, you know, you’re always have your hands in a pot and you’re a tagline on your LinkedIn was like, I like to build things, which I think is great.
Joshua Miller: Thanks. Yeah. I mean, I’ve always been specifically, in computer vision, right? So like the automatic analysis of images. And so my first company this is going to sound super weird was in satellite imaging for farms. Right. So what we were doing was looking at satellite images and seeing like, could you look at 100 acres of corn and find a disease, right? In that field tell the farmer. That sounds very far from medical imaging. The same computer science problem as finding a lung nodule in a CT scan. Everything that I do kind of looks weird, right? It doesn’t seem that connected, but it’s all got that theme of this kind of part of computer science that I love.
Jennifer Callahan: So let’s go back then to Gradient Health, obviously, because that’s what we’re really here to talk about. Say, I’m a company who’s coming to you, and I have this AI software that I’m trying to develop, and I need to access certain types of, let’s say, liver images because we’re looking at liver cancer or liver diseases. We set up. And, you know, I get access to that. Obviously, there’s a database. Is it like a normal like, say like a Google search where you’re just typing in the word like liver or different parts of the liver?
Joshua Miller: Yeah, it’s a little bit smarter than that because you have to ask for certain things. Right? You might say like, I really want liver screening, but I want specifically liver CT scans, and I want the slice thickness to be less than, let’s say, three millimeters. It tends to be a little bit more numerical and quantitative than you’d imagine a Google search, right? When people are building these algorithms. And so we have this interface where you can log in, do type in something like liver screening. Right. But then you also configure all these other parameters for the data you want. And it goes into the database comes back and then you have your data set. They hold on to those images for a certain amount of time. They use it to essentially teach the AI what different diseases look like. And then at the end of the contract, they remove those images or delete them, right? In some cases, they’re doing a regulatory filing. So they’re trying to show the FDA, look, you know, I built this algorithm. You should approve it. Here’s all my data that shows that it actually works. We actually got a grant from the FDA slash NIH to make these kinds of data sets, right, that are for proving your algorithm as a medical device.
Jennifer Callahan: For a company like that, who’s going through this? Is it suggested that they have a certain amount of images that they’ve used to develop the software?
Joshua Miller: Heavily depends on what you’re claiming the AI can do, right? So if you’re saying and it’s always thought about in terms of risk, right? So let’s talk about the stroke cases that we were talking about before. If you move a case to the top of that stack and it turns out the person doesn’t have a stroke, there’s not a lot of consequence to that. Their image was going to get red anyway, right? What is? Let’s say a higher bar is you say someone has cancer with an eye and they don’t. That is extremely negative. And so the FDA will ask for higher and higher bars of proof depending on what you’re claiming you can do. Can you potentially suggest there’s a stroke, not a super high bar? Are you saying someone definitively has cancer? Yeah, that’s a very high bar. Right. And so that could mean, look, you only need 300 images if you’re claiming A and you might need 10,000 if you’re claiming B. Once someone explains to us what they’re trying to build, we can generally give them some advice on how much of a data set they need.
Jennifer Callahan: And I guess, in terms of different types of medical conditions or diseases, obviously, I feel like with like cancer, a lot of times it’s they want to take a biopsy of whatever the area is to give that definite answer, but it might be a body part that really doing a biopsy kind of really isn’t an option.
Joshua Miller: That’s a good example. And so you don’t want to do one unnecessarily. If it’s pretty unlikely someone has cancer, you want to make sure you don’t do it. And if it’s very possible someone has cancer, you want to make sure you do it. And so we look at things like when you’re scoring lung nodules, there’s this thing called the lung rad scale, right where you say, oh it looks like lung rads one lung rads two basically increasing up to number four, where number four is like, well, this is probably cancer. I can get a lot more granular than one, two, three, four. It can be said, this is precisely a 67% chance of cancer. It’ll never be able to definitively say yes or no, but it can help the physician make decisions in their care of the patient.
Jennifer Callahan: Software like this is almost like The Amazing Race. How you said, like, there’s so many different companies who are trying to develop software like this that, you know, you’re just hearing about it all the time. And so almost like it’s become like common stance, I think.
Joshua Miller: I agree with that. I would have agreed with that like two years ago. Right. Yeah. Tons of companies that were coming out that all kind of did the same thing. Right. It was all like, oh, I can find your lung nodules or whatever it might be. At this year’s Rsna in Chicago, I saw some things that blew my mind and made me very convinced that the next two years are going to be very different from what the past two years were, one of the demonstrations that basically the ChatGPT of radiology, right? If you’ve played around with it, it had a chest x-ray up there, right, and a box where you could type in and you could ask questions. You could say, give me a summary of what’s wrong with this chest x-ray. And it would read the chest x-ray and write a few snippets about what was going on. Then I got to ask it and I was like, okay, type in the box. How would I explain this to the patient’s partner? And it even gave like a little bit of advice and I was like, you do this. And then the guy at the booth, it was this company called Hopper. Hopper said, do you know any other languages? And so I started talking to it in Spanish and it started answering in Spanish. Right? For a patient, I think that you’re right in saying a lot of the stuff that came out in the last five years was kind of par for the course. I think what you’re going to see come out in the next two years is going to be a fundamental shift from that.
Jennifer Callahan: So speaking of the future, I mean, obviously you’ve done a lot of developing your company. Could you possibly share where you think Gradient Health currently might be going, or are you working on something particular, or would you rather not share?
Joshua Miller: I mean, I didn’t come to the interview to shut up, right? What I see happening a lot is it used to be that the typical AI that people were building in space, you needed like a thousand images to build that AI. To build things like what I just described, you know, kind of what’s called a foundation model in the space. You need a million chest X-rays, which is not simple or easy by any means to come by. That’s what I’m pretty excited about for Gradient. It’s like it used to be these one-off data sets from one hospital for a thousand studies that you’d build these algorithms on, but you end up with these kinds of not diverse data sets. They don’t come from multiple types of machines, etc., and they don’t end up at the scale. You kind of need to build these. Again, they’re called foundation models. That’s what I’m most excited about is provisioning those kinds of data sets that will enable those kinds of algorithms.
Jennifer Callahan: What’s the amount of time that goes into something like that? I guess a foreseeable game plan of when you would like to have something completed, or how long you think it would take you?
Joshua Miller: I mean, we’re already doing that stuff today. Okay, that’s more like I want to do more of it because I kind of see that as the future of development in this space.
Jennifer Callahan: What kind of advice do you give to people out there who have, you know, spirit like you?
Joshua Miller: I think it’s. Just to get started. Right? And that sounds really dumb, right? It sounds really simple and it sounds like a major oversimplification, but. I think a lot of people will wait for a perfect idea that never comes. Whereas every successful business I’ve ever seen started out as an “okay idea.” They started doing it. They learned a lot and it became a great idea. And so the most kind of fundamentally important thing you can do is just get started. You don’t have to wait for that perfect idea. Do the thing that sounds good enough, and you’ll learn enough to turn that into the thing that is the perfect idea.
Jennifer Callahan: Well, this was an awesome conversation, everybody. This is Josh Miller sharing his wonderful company with us, Gradient Health. Can’t wait to see what you were saying with the Cessna. You know, with the future of AI that’s coming out. And I’m sure that you’re a part of helping the development of that. So thank you for taking the time with me tonight. I really appreciate it.
Joshua Miller: Thanks for having me.
Jennifer Callahan: All right, everybody, make sure you listen in on the skeleton crew, you know, past and future episodes. I’m Jen Callahan here with Josh Miller. So we’ll see you guys later.
Jennifer Callahan: You’ve been listening to the Skeleton Crew, brought to you by xraytech.org, the Rad Tech Career Resource. In the next episode, join us to explore the present and the future of the Rad Tech career and the field of radiology.