From Practice to Launch: Building an AI Rad Tech Company, with Rad AI Co-Founder Jeff Chang
Episode Overview
- Episode Topic: In this podcast episode, we delve into the integration of AI in radiology with Jeff, co-founder of Rad AI, exploring its transformative impact on radiology workflows and patient care. Jeff also shares his firsthand experiences that X Ray Tech can learn from, juggling entrepreneurship with his role as an ER Radiologist, supervising across multiple hospitals. We finally explore the future of radiology in an AI-centric world, discussing industry trends, job opportunities, and essential advice for radiographers navigating this evolving landscape.
- Lessons You’ll Learn: You’ll discover how AI can transform the health sector, particularly radiology, and its potential to combat practitioner burnout and improve patient care. Jeff provides insights into how Rad AI makes X-Ray Tech lifes easier by enhancing radiology workflow, automating report generation, and customizing to individual radiologist language preferences. You’ll also learn about the challenges and triumphs of launching an AI-based health tech startup and the importance of continuous learning and staying abreast of technological advancements in the healthcare industry.
- About Our Guest: We welcome Jeff Chang, ER radiologist and Rad AI co-founder. At 16, Jeff entered a medical school and became the youngest radiologist in the U.S., specializing in musculoskeletal MRI. During his career, he developed an interest in machine learning, leading him to found Rad AI in 2018. The company aims to boost radiology efficiency and improve patient care through innovative AI solutions.
- Topics Covered: During this enlightening episode, we discuss Jeff’s inspiring journey from his early medical years to his overnight shift experiences as a radiologist. We uncover the conception and unique features of Rad AI, focusing on how it automates dictation and customizes to individual radiologists’ language. We also explore the impact of Rad AI on radiology language, workflow, and follow-up care coordination. Furthermore, we delve into user feedback (in particular X Ray Tech), implementation challenges, and future developments. This comprehensive conversation offers invaluable insights into the world of AI-powered radiology, bridging technology and healthcare in an unprecedented way.
Our Guest: Jeff Chang, Co-Founder of Rad AI
Our guest for today’s episode, Jeff Chang, brings a remarkable wealth of knowledge from his work in the rapidly advancing field of AI and radiology. He is a co-founder of Rad AI, a company that seeks to improve the workflow within the sphere of radiology, employing cutting-edge technology as its backbone.
Jeff embarked on his academic journey at an incredibly young age. He was just 13 when he began college, and three years later, he entered medical school. This fast-paced path led him to become the youngest radiologist in the United States. His specialization is musculoskeletal MRI, a complex imaging technique that examines bones, muscles, and related structures.
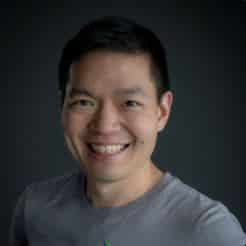
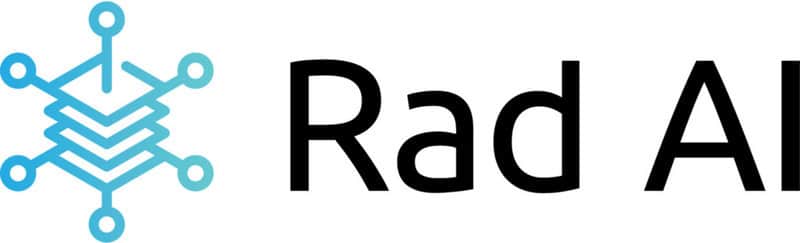
Jeff’s unique work experiences set the stage for the inception of Rad AI. Most of his time at Greensboro Radiology was spent working during the night. These intense overnight shifts, although challenging, provided him with invaluable insights into the profession’s struggles. From these experiences, Jeff understood the importance of efficiency, quality reporting, and proper patient follow-up in radiology.
Driven by his unique insights, Jeff co-founded Rad AI. The company’s primary goal is to solve the many issues that radiologists face daily. Rad AI concentrates on developing tools that save time, reduce professional burnout, improve the quality of reports, and ensure efficient patient follow-up.
Throughout this journey, Jeff has remained committed to creating a better working environment for radiologists. His efforts, along with those of his team at Rad AI, continue to impact the field of radiology in the most positive ways. We are thrilled to have him on our episode, ready to share his journey, the inner workings of Rad AI, and its pivotal role in the evolution of radiology.
Episode Transcript
Jeff Chang: [00:00:00]
Hiring is one of the most important things for startups. Even one really great person can change your culture for the better and make your team far more productive and efficient. And then one bad person or the wrong person for the company can completely change the culture as well.
Jen Callahan: [00:00:20]
Welcome to the Skeleton Crew. I’m your host, Jen Callahan, a technologist with ten-plus years of experience. In each episode, we will explore the fast-paced, ever-changing, sometimes completely crazy field of radiology. We will speak to technologists from all different modalities about their careers and education. The educators and leaders who are shaping the field today and the business executives whose innovations are paving the future of radiology. This episode is brought to you by X-raytechnicianschools.com. If you’re considering a career in X-ray, visit X-raytechnicianschools.com To explore schools and to get honest information on career paths, salaries, and degree options. Hey, everybody. Thanks for being with us here tonight. We have a really special guest with us. His name is Jeff Chang. He is an E.R. radiologist, turned into entrepreneur who has co-founded the company called Rad AI. He was working with a company of Greensboro excuse me, not company. He was working for Greensboro Radiology and has transitioned from about 2020 into this new company that he himself and Dr. Gerson have developed. So, Jeff, thanks so much for being with us today.
Jeff Chang: [00:01:38]
Thanks for having me.
Jen Callahan: [00:01:39]
So I just gave a little bit of an intro of you, but do you want to give a little bit more background of yourself and then how you and Dr. Gerson ended up developing this company?
Jeff Chang: [00:01:50]
Sure. I’m Jeff and I started really young, went to college at 13, med school at 16, became the youngest radiologist, and second youngest doc on record in the US. So did a fellowship in Musculoskeletal MRI, but then ended up practicing ER radiology, doing mostly all or essentially all overnight shifts for ten years with Greensboro Radiology. I did that mostly to have more time to be able to explore other areas. So I found a really part-time role. So that allowed me to just do overnight shifts so that I could then go to business school, do some graduate work, machine learning. And then we started Rad AI in 2018, really focused on improving radiology workflow. So how do we save radiologists time, reduce fatigue and burnout, improve the quality of radiology reports, and also improve the follow-up for patients making sure that they get their follow-up care?
Jen Callahan: [00:02:41]
Yeah. So one, we need to touch on a bunch of different stuff that you just talked about. One, the fact that you became a doctor when you’re 16, I’m sure everyone that you ever tell that their mouth just drops. I don’t know if you remember from back in the 90s, the TV show Doogie Howser, MD but I always try to go on whoever I’m having on the show as a guest. I like to go in and do a little background to see who I’ll be speaking with. And as soon as I heard you say like 13 and 16, Doogie Howser totally popped into my mind. So and then also, too, are you doing overnight shifts as a radiologist? So what type of like shift work was that for you? Is that like a normal 7 p.m. to 7 a.m. type? How almost like a nurse would work?
Jeff Chang: [00:03:21]
Yeah, it was 9 p.m. to 7 a.m. So I would fly to North Carolina for one, two, or occasionally three weeks at a time and just do seven nights, 14 nights, 21 nights in a row. It was moderately busy. So generally probably averages somewhere between 150 to 200 studies a night.
Jen Callahan: [00:03:39]
Wow. I mean, I can understand why you would want to develop a company like what you did. I mean, was it solely just yourself, or did you have anyone else reading with you?
Jeff Chang: [00:03:48]
No, I mean, we usually had more than one person covering overnight. It depended. So when I first started Greensboro, radiology was only about 30 rads. And so I was the first overnight hire. And so for, I think like six hours of that time each night, I was on my own. But then after that, over time, we hired more people because the group got larger, there was more coverage, and so on.
Jen Callahan: [00:04:08]
So then when you’re working that overnight shift, you were solely based, like you said, E.R. radiology, mostly just reading whatever, like Cat scans and like pretty much like x-rays. And I know usually sometimes they might do like a stat ultrasound, but I feel like there you’re probably your three modalities that are being read at that time of night. Would you have to read anything from that was like left over from the day that maybe the radiologist hadn’t gotten to?
Jeff Chang: [00:04:31]
If there was stuff that needed to be caught up, especially like outpatient imaging that sometimes the group had a lot of extra volume to read. So mostly EBCT is X-rays, ultrasound, but then also some inpatient stats and so on. Very rare MRIs. Yeah.
Jen Callahan: [00:04:47]
From working in that type of environment where you were reading so much, is that where everything started churning? Like there has to be a better way to do this so that we’re not having so much on our plate?
Jeff Chang: [00:05:00]
I think for me, it was I gradually became interested in building more of a technical background. So I went to Dev Bootcamp for Ruby on Rails JavaScript in San Francisco. That’s where my co-founder and I met and then went to University of Edinburgh to do some graduate work on machine learning. And I think from there kind of figured out that we could apply AI machine learning to radiology to help radiologists. But our focus has always been on reducing fatigue and burnout, on saving radiologists time. When you think about what radiologists do on a daily basis, we spend 75% of our time dictating reports. And so our first product helped automate that process, streamline a lot of the manual dictation that radiologists do save about an hour per nine-hour shift.
Jen Callahan: [00:05:41]
Okay. So spoke to someone like a week or so ago who was an AI company as well, but their technology or platform was different than I feel what your website is showing. So theirs was talking about, you know, they’re looking at an image and spotting something that maybe a radiologist might not pick up on. That one is like helping with the report, but yours is almost, like you said, generating a whole report. Now, is this being based on what they would normally dictate?
Jeff Chang: [00:06:07]
Yeah. So we’re not doing interpretation at all. We’re purely on the operational side of radiology. Most AI companies in the space are working on the imaging side, which requires FDA clearance. For us, we’re actually purely doing text. So based on what the radiologist has already said, based on what they’ve already seen and they dictate what they see. And then we use that text to generate just the impression was our first product. So the summarization conclusions of the radiologist. And so from the 21st Century Cures Act, the FDA determined that that’s not considered a medical device anymore. Much more straightforward. But also at the same time, it’s a very difficult thing to do well, because you have to be customized to each individual radiologist’s language because radiologists do care a lot about their language. It’s part of what we consider the art of radiology, especially the impression section.
Jen Callahan: [00:06:52]
Which is your radiology language? Are there certain terms that you use that you feel like you use more or that you use to use in the past?
Jeff Chang: [00:06:59]
I tend to be more systematic about language in radiology reports than most. But I mean, at this point, having seen reports from thousands and thousands of radiologists, you see such a huge variety across every radiologist. It just depends on where we’ve been trained. We got used to and kind of changes over time as well.
Jen Callahan: [00:07:18]
Do you find like different regions of say, the United States use different verbiage?
Jeff Chang: [00:07:23]
No, I would say it’s mostly dependent on where you trained. So specific radiologist, attendings that you had in your training program, but then also kind of taking up habits from other whether your other group partners or other people that you’ve worked with. We usually are a combination of all the different radiologists that we work with in the past. So.
Jen Callahan: [00:07:42]
Okay. All right. Yeah. So you’re like a melting pot of us.
Jeff Chang: [00:07:45]
I would say most radiologists tend to pick things up from their training programs and from specific radiologists that they work with and attendings that train them and so on. And that usually explains kind of all of our different habits or many of our different habits.
Jen Callahan: [00:07:59]
All right. So the automated generated report. Is it like the radiologist is you know, they do partial of the dictation, and then the technology finishes out like you said, the summary or what the impression is at the end? Okay.
Jeff Chang: [00:08:12]
Yeah.
Jen Callahan: [00:08:13]
That’s awesome. That makes sense. Now, I was like trying to exactly figure it out. So like, doctors actually sitting there reading, they’re looking through the whole entire imaging as they’re looking at a chest x-ray. They’re looking at the lungs, the heart. They’re actually, you know, addressing each one. But they don’t have to sit there and do the final portion of what the impression is. Or for someone who isn’t familiar with radiology reports, it’s basically like the summary of what has been said inside that report. Correct?
Jeff Chang: [00:08:38]
Yeah. So generally we find it reduces the number of words that a radiologist has to say by about a third every day and of course, gives a bit of a cognitive break. So it helps reduce fatigue and burnout and so over time, continues to build out more products across the radiology workflow. So our second product helps with ensuring follow-up care coordination, so identifying any incidental findings that require follow-up in the radiology report. So this is after it’s been signed off. So the final report, knowing what the follow-up timeframe should be, knowing what the consensus guidelines are and so what the correct studies are, and then automating the patient-provider communication and then automatically tracking when the patient gets scheduled for the exam and so on and so forth, and finally gets the exam done. That way we can increase the follow-up rates for patients to pass 90% usually.
Jen Callahan: [00:09:22]
So the report then can automatically figure that out based on what the impression was?
Jeff Chang: [00:09:28]
Oh, no, just based on anything that was mentioned in the report for a recommendation. Essentially, we can also pick up on incidental findings or significant findings like potential cancers that didn’t have a recommendation, but we usually default to whatever the radiologist said. Right. So whenever the radiologist recommended some sort of follow-up procedure, we can automatically pick up on that. And there’s no change to the radiologist at all in terms of their workflow. So it’s just based on the final report. And because at this point, we work with over 350 million reports from all of our customers across the US. So we have a lot of understanding of how different radiologists dictate their recommendations and so on.
Jen Callahan: [00:10:02]
So when like, say, a health system decides to go with your company to set this up, does each radiologist have to sit down and do a certain amount of dictation so that the technology can pick up on it or?
Jeff Chang: [00:10:15]
No, no, you’re saying first, product for impression generation? Yeah. Yeah. So we work with the historical reports of the radiologist, usually about five years of historical reports. That’s all pre-trained beforehand. So as soon as the product is rolled out, it’s already been customized to each radiologist.
Jen Callahan: [00:10:30]
And nothing that they need to click to have that done. Like, you know, the software’s already installed. So as soon as they’ve done their reading, the impression just automatically generates at the end of the report.
Jeff Chang: [00:10:40]
Yeah, we’ve made it as quick as possible essentially. So when auto mode is turned on, as soon as you get to the impression it detects where you are in the report. So it automatically generates the first time you get there. The goal for designing products that work well for radiology workflow is you really have to make it zero clicks, so you have to make it, no change to the existing workflow. So there’s nothing new to learn and so on.
Jen Callahan: [00:11:01]
Yeah. I can only imagine like decreasing what at least an impression could be. What, maybe like 100 words, give or take.
Jeff Chang: [00:11:07]
It varies a lot. Yeah.
Jen Callahan: [00:11:08]
Decreasing that thought out of out of what you’re saying. If you talk a lot or if you talk more than you normally would I mean, it makes you tired.
Jeff Chang: [00:11:16]
Yeah, it does. Now we can also because we do track the metrics on how many words it saved you and so on. So we can see over the course of this year, how many times would you have read like The Lord of the Rings cover to cover? So you saved all of these words.
Jen Callahan: [00:11:32]
So you were talking about the different platforms that you have with the radar. Do you have the Omni, which is what we’re talking about, that generates the impression, the continuity, which does the follow-up? And then there was another one on your page, which is the Nexus. Can you just explain what that platform is?
Jeff Chang: [00:11:46]
Sure. Nexus is a smart work list for radiology groups. As a radiology group, when you when the radiology group gets larger and you have thousands or tens of thousands of studies coming in every day, you can’t have everyone read off the same work list just because there is different hospital credentialing, there’s different subspecialties, different SLAs for different specific sites. Particular outpatient providers might only want specific radiologists to read their studies as a dynamic workload that we automatically determine, based on all of these rules, which radiologist gets the right study at the right time. And over time we’re adding more driven elements to that as well. So we acquired Intelligence Doctor Bill Boone and Doctor Woojin Kim start up last November and so are in the process of integrating forecast into Nexus. So that’s demand forecasting for radiologists, knowing what kinds of studies are coming in at what time, both in advance and also real-time. And then knowing how much coverage you currently have, do you need additional staffing? But then can you also distribute the cases in the right order for each radiologist to be most efficient, but also enjoy the process of reading less burnt out, less fatigued.
Jen Callahan: [00:12:47]
For the forecasting? Could almost be like in the winter you might expect to have more chest X-rays because of cold and flu season.
Jeff Chang: [00:12:54]
So for specific days of the week and specific days of the month based on weather patterns, based on your historical trends, knowing what study mix is going to be coming in each day, most likely that can be predicted out, eight weeks in advance, but also in real-time day of seeing when a bunch of orders have just hit the system. And so you know when they’re going to be scanned. And so then when you need additional coverage, you know, you can you can set automatic alerts, alerts for the radiologists, and so on. So can everybody help pitch in at this time? But then also for some groups say, for example, they know that they have this pending X-ray volume and they need to make sure that everybody picks up on their amount of the workflow. Then you can also have kind of a mixed push-pull system where, say, for example, there’s 100 X-rays to be read and ten radiologists that can read them. Then you can push ten to each radiologist, right? And to fit that as part of their workflow so they can still pick some studies, but then also make sure that they get through the required volume.
Jen Callahan: [00:13:47]
Okay, that’s cool. So you’ve had this implemented like ten of the largest radiology practices in the United States.
Jeff Chang: [00:13:56]
Eight of the ten largest radiology practices at this point? Yeah. We work with over 100 radiology practices, health systems across the country now in use by radiology groups, health systems that comprise just over 30% of all medical imaging in the US.
Jen Callahan: [00:14:11]
So does your alma mater of Greensboro Radiology. Do they use Rad AI?
Jeff Chang: [00:14:16]
Yeah, yeah. So they also use their part of RP now.
Jen Callahan: [00:14:20]
To bring this on when you were developing. Did you have to go to different lot to, say, use someone as a guinea pig? Because that sounds really wrong. So you were a radiologist, say, at Greensboro Radiology. You know, did you go to them and say, would you mind if we tested out this software?.
Jeff Chang: [00:14:34]
At the very, very beginning before it was ready to expand more broadly, I was the first user and tester and seeing how well it worked and so on and so forth. And we made a lot of fixes and improvements over time. The thing about our product is that it always has radiologists to review, so it’s never the result that goes out directly, right? The radiologist is always reviewing the result and making their changes. There’s always that final check by the radiologist.
Jen Callahan: [00:15:02]
Sure. Yeah. I mean, definitely has to have like a check and checks and balances, you know, with anything like that, especially in terms of health care. So on your team that you have developing this software yourself as a radiologist, obviously, do you have other radiologists that have been with you developing the technology or the software?
Jeff Chang: [00:15:20]
Yeah, the number of radiologists on our team has actually grown significantly over time. At this point, I mentioned equity intelligence, which we acquired in November. Dr. Bill Boone and Dr. Woojin Kim both joined the executive team as CMO and CMO. So we actually have three really well-known radiologists now that are on the executive team. They were both Co-CEO of Nuance for several years before they started their own startup again.
Jen Callahan: [00:15:45]
Do you think that, with the implementation of this type of software within like different healthcare systems, obviously it changes the workflow for the radiologists, but do you think it affects anywhere else within like the radiology department? Does it help at all, say, the technologists who are performing the exams?
Jeff Chang: [00:16:01]
Yeah. So one of the things that was working on was optimization of technologists’ schedules. So that was a separate product within their resource product. So essentially when you look at the typical scanner schedule, there are certain studies that, you know, will take longer or shorter or patients may not be there or so on and so forth. So you could move those around to fill in gaps, improve utilization for the scanner. But also one thing that health systems have started requesting from us is whether you can route the technologist in a better way. So say, for example, if you have a health system with radiologists or sorry, the technologist has to go do portable ICU films, then knowing all the studies that need to be done in a certain area before they have to kind of move around the hospital and then also knowing when specific stats are coming in so that there’s more a better alerting of that. So being able to reprioritize the scanner accordingly. And then finally, also being able to provide a list of this is the schedule and the order in which your patients will be gotten to so that nurses won’t keep calling technologists about pending orders. That last part is not something that we built yet, but it’s likely something that we’ll be working on over the next year.
Jen Callahan: [00:17:15]
Do you feel like you met any challenges along your journey of building this software?
Jeff Chang: [00:17:22]
There’s been a lot of ups and downs over the years, so part of running a startup, especially when it’s smaller, is that you hit upon existential threats throughout the course of early startup life. So any single day can have very high ups and very low lows. I think as the company has gotten larger, that becomes less the case, right? So at this point, the company is significantly larger and we have employees in 23 states, so it’s pretty spread out. Now. Early on, there were definitely a lot of.
Jen Callahan: [00:17:51]
I’m sure.
Jeff Chang: [00:17:52]
Different types of words. A lot of it really comes down to having the right people on the team. Hiring is one of the most important things for startups. Even one really great person can change your culture for the better and make your team far more productive and efficient. And then one bad person or the wrong person for the company can completely change the culture as well. So yeah, and they might not be like that. So people aren’t good or bad overall. They’re more like, not right for your particular culture. You have to find people that are a good mutual fit.
Jen Callahan: [00:18:22]
Or maybe not even the right fit for the position that they were originally hired for sure. You know, so speaking of that, people that you hire, are there certain backgrounds that you look for in the people that you hire? You know, are you looking solely not solely, but like maybe specifically for people who mostly have a computer background, computer technology or obviously you said you do have radiologists who are on board on your team. But I guess depending on what your position is hiring.
Jeff Chang: [00:18:51]
It’s across the board, I would say, right now. So I am still more than half engineering and we can always use additional engineering development talent. So we are currently looking for full stack developers as well as other engineers, machine learning engineers, so on and so forth. We’re also hiring on the customer success side. So making sure that we have strong customer support and customer success managers and to some extent on health system sales and strategic partnerships, some roles in the operations. Site as well. So there’s a lot of different available, so different types of. Candidates for each of those.
Jen Callahan: [00:19:27]
So you said the forecasting is something that you’re in the. Midst of developing. Are there any other ideas that you have out there on the table for the future? What you’re looking to develop? So we have.
Jeff Chang: [00:19:38]
An additional product that is being announced later this year and then further products beyond that that we’re working on. So mostly just centered around the radiology workflow. So how do we provide it or streamline it further? How do we provide radiologists more time savings and kind of expand beyond the radiologists? How do we help technologists as well and so on and so forth? So a lot of different ideas and it’s all a question of prioritization, right? So there’s only a limited number of things that you can work on given the size of the team and the specific needs of the market.
Jen Callahan: [00:20:11]
Do you ever get any feedback or critique from the different radiologists that use the program? And do you take that into consideration in terms of further development?
Jeff Chang: [00:20:19]
Yeah. So in our omni application, for example, after every case you get an optional feedback window where you can write a comment and give it a thumbs up, thumbs down, it’s completely optional. But if you do, that’s also the fastest way for us to make changes. So we have a customer success team that reviews all of that, the comment feedback every day, including both customer success managers and also radiologists on our team. And then you can also submit support requests directly through that or new feedback for new feature ideas, and so on, and also can request a response directly from the customer success team.
Jen Callahan: [00:20:50]
Let’s focus on you for the last couple of minutes that we have here. You’re in the hot seat. First, let’s start. What’s your favorite modality? Do you like X-ray MRI, Cat scan, ultrasound?
Jeff Chang: [00:21:02]
Having done ten years of radiology, I’m x-rays. Cts are all very straightforward or tend to be especially ed overnight type volume. I would say getting an EMR is still fun because I did my fellowship in musculoskeletal MRI. It feels like a change of pace more than anything else. And because in fellowship we did far more knees than any other joint. So it’s also something that feels very familiar.
Jen Callahan: [00:21:28]
Okay, so you like knee MRIs? If you had to choose an X-ray study, what would you choose?
Jeff Chang: [00:21:34]
Maybe a knee X-ray or a chest X-ray?
Jen Callahan: [00:21:36]
Okay, How about a Cat scan?
Jeff Chang: [00:21:38]
Just has more variety and complexity than most other types of radiographs in terms of variety of findings and diagnoses. Knees just because there’s all these different interesting pathologies and most of the fractures are not as subtle as might be seen on like ankles or something.
Jen Callahan: [00:21:57]
So in your years of being an overnight radiologist, is there anything that ever stood out to you from memory that was a crazy case or that was something crazy that I read?
Jeff Chang: [00:22:09]
Of course, you see a lot of different things over the years. There’s never any shortage, of course, of things inside people’s bodies that they inserted.
Jen Callahan: [00:22:18]
Very true. Very true.
Jeff Chang: [00:22:19]
From that. I mean.
Jen Callahan: [00:22:21]
Is your hospital a trauma hospital? Oh.
Jeff Chang: [00:22:23]
Yeah. It was a level three trauma center.
Jen Callahan: [00:22:25]
Level two.
Jeff Chang: [00:22:26]
Yep.
Jen Callahan: [00:22:27]
Okay.
Jeff Chang: [00:22:28]
So, yeah, we had our lots and lots of motor vehicle accidents. More than anything else, I’d say gunshot wounds in Greensboro.
Jen Callahan: [00:22:34]
Is that by like Charlotte? I’m not I hate to say it’s about.
Jeff Chang: [00:22:38]
An hour and a half from Charlotte, so it’s just over halfway between Charlotte and Raleigh Durham.
Jen Callahan: [00:22:44]
And that’s crazy. So you used to you would commute from the San Francisco area to Greensboro?
Jeff Chang: [00:22:50]
No, When I first started, I was actually in LA, so I did fellowship in business school. In LA, I was still in business school because I was doing the fully employed program at UCLA. And so I found Greensboro Radiology, which had just over 1 in 4 weeks. So I would fly over for a week of nights and then I would come back. So overall did just over 1200 overnight shifts.
Jen Callahan: [00:23:10]
All right. So as a final word, do you have a piece of advice that you would give to any radiologists or radiographers that are out there in terms of the field that they’re in?
Jeff Chang: [00:23:19]
I would say keep learning, keep apprised of the newest and latest technologies, and see what might work for you, your health system, your practice. There’s always new technologies and new software that can provide efficiencies and gains. And then, of course, some things that don’t work. So when you learn more about whether machine learning or about software generally, so on and so forth, you have a better eye at seeing what might work or what might not and also keep life interesting, right?
Jen Callahan: [00:23:49]
So I just learned about the software myself within this past month. And now that I know that the software can span like many different genres, you know what I mean? Like you’re doing the techs. I was kind of just like, wow, it’s crazy. All right, everybody, this is Jeff Chang. He joined us tonight from the San Francisco Bay Area. I’m so honored that he took the time to meet with me tonight. And I can’t wait to hear more about what you guys have in development. So thank you so much.
Jeff Chang: [00:24:19]
Yeah, thanks for having me.
Speaker3: [00:24:26]
You’ve been listening to the skeleton crew brought to you by X-ray technician Schools.com. Join us on the next episode to explore the present and the future of the rad tech career and the field of radiology.